Real-Time ESG Data Management
ESG (Environmental, Social, and Governance) data collection and reporting has become a corporate priority, with over 96% of S&P 500 companies publishing sustainability reports in 2021, according to research from the Governance and Accountability Institute.
There are several factors driving the adoption and use of ESG data; ranging from consumer preference for companies with positive ESG information, to employees, who increasingly believe environmental, social, and governance metrics are important indicators when choosing an employer.
Many government bodies and regulators either have, or are considering, mandatory ESG data collection and ESG data reporting requirements for corporations under their jurisdiction. The European Union is taking the lead here, with several key pieces of legislation either already enacted, or coming soon. In the US, the SEC has also announced proposed rule changes for securities reporting, mandating companies make detailed climate-related disclosures in their filings.
In addition to companies that report on their own data, financial firms, including the private equity industry, use ESG data and research to weigh risks and identify opportunities for the companies they invest in.
Faced with growing scrutiny around ESG reporting and scoring, companies are struggling to meet ever more detailed and comprehensive reporting requirements. At the heart of the problem is the sheer volume and variety of data companies are expected to ingest and analyze to produce the scores that investors, consumers, and government entities demand. And with real-time data making its way into reports, ESG data management is becoming even harder.
ESG data collection and analysis
The volume and variety of ESG data makes collection and analysis difficult. The data collection problem can be broken down as follows:
Variety
Unlike financial datasets, which are mostly numerical, ESG metrics can include both structured and unstructured datasets, like an email or a media report. If a company wants to analyze satellite data to derive their own climate dataset, they may even need to analyze images and videos. Given these variables, companies need to employ a data model that can support many different types of data.
Velocity
As companies increasingly integrate real-time data sources into their ESG scoring systems, the velocity of data collected and analyzed increases exponentially. One example is loan due diligence in the financial sector. As customers demand faster loan approval turnaround times, financial institutions that currently rely on quarterly ESG data to make those decisions now need the information in real-time to instantly approve loans in an ESG compliant manner.
Volume
The increased variety of data sources, coupled with the growing velocity of data being collected leads to an increase in the sheer volume of data requiring analysis. Currently, ESG ratings and scores are derived from a blend of human judgment and model driven quantitative rating. But as the volume of data increases, along with the need for instant analysis of that data, real-time analytics and an increased use of AI/ML tools will become an ever greater part of ESG ratings and reporting.
On top of this, there are also no universally applicable ESG standards, leaving companies having to deal with multiple different standards, with different data requirements, depending on which jurisdictions they operate in.
Real-time ESG data analytics
Companies are increasingly incorporating real-time data into their ESG analysis, reporting, and scoring. Harnessing technologies such as cloud computing, AI, and machine learning, those that utilize real-time data can, for instance, instantly parse breaking news stories for ESG-related data on their investments, or incorporate up-to-the-minute satellite data into reports on a firm’s environmental impact.
The financial services industry in particular is taking a lead on integrating real-time ESG data into investment decisions.
Asset and fund managers use real-time data platforms that allow them to calculate up-to-date ESG scores to aid investment decisions and risk calculations. For example, a bank looking to invest in an electric vehicle company would be alerted to a breaking news story about a hazardous accident at the manufacturer’s battery plant, with follow up data from social media or analyst reports quantifying the size of the public reaction and the level of negative market sentiment around the accident.
MongoDB and ESG data management
MongoDB Atlas is an ideal data foundation for ESG platforms. MongoDB Atlas uses the document data model, giving users the ability to ingest data from almost any source, consolidate data from a number of siloed data sets, enable the easy search of that data, and with a few clicks, create customized views of the data without the need for additional ETL operations to other databases or tools.
MongoDB Atlas also future-proofs your ESG data platform with a flexible data schema that can easily adapt to rapidly changing ESG requirements and standards.
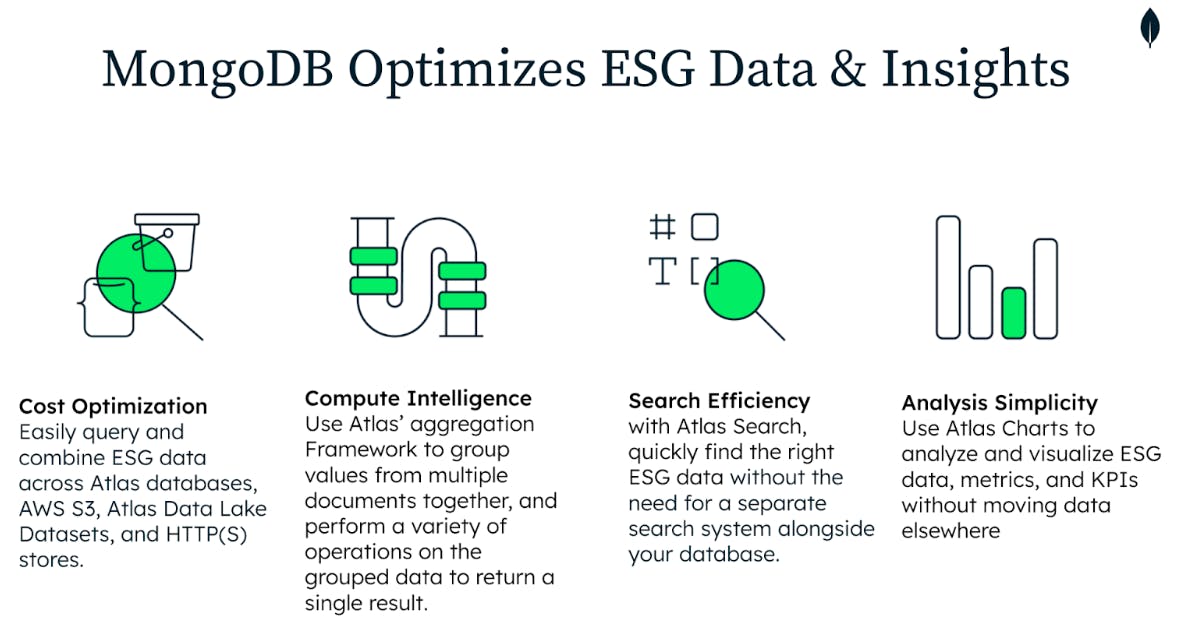
See why Hydrus chose MongoDB Atlas as the basis for its ESG reporting platform.
FAQ
ESG data definition
ESG (Environment, Social, and Governance) data comes from a growing list of sources, all of which help “score” a corporation based on how well positioned it is to handle the risks and opportunities presented by the environment, societal stakeholders, and corporate governance.
Environment - What are a company's greenhouse gas emissions? How about its stewardship over natural resources? And how well positioned is it to weather physical climate risks, like global warming, flooding, drought, fire etc.
Social - How does a company measure up against prevailing fair wage and employee engagement metrics? What impact does a company have on the communities where it operates?
Governance - How well is a company managed? How responsive is a company to shareholders? How accountable is leadership? What safeguards are in place to ensure transparency?
The growing interest around ESG data science and data analytics has prompted the rise of a new industry of ESG data companies and ESG data management software vendors.
What are the different ESG data sources?
ESG data may come from two primary sources; 'inside-out' and 'outside-in'.
Inside-out data is supplied by companies, used for analysis, and usually lags 6-12 months due to annual ESG-related disclosures.
Outside-in data is more regularly updated, sometimes even in real time. Most financial institutions, including banks who often have access to a lot of financial and company data from their customers, do not rely solely on their own data. ESG data analysis requires a broad range of inputs and data that the bank does not possess or can obtain even from their customers.
For example, a bank may want to assess the risk of flooding for a chip manufacturing company that has factories in several provinces in China. The bank would need to collect the flood data from the different operating locations in order to score the risk.
As banks don’t typically collect flood data themselves, the bank would purchase data from third-party climate data vendors. At this nascent stage of climate risk assessment within the banking industry, it is likely that the bank would not even attempt to collect the raw climate data and create the risk models to score the risk, relying instead on third-party risk scoring vendors.
The bank would then make use of these scores and combine in models which they have strong competencies eg. credit risk to come up with flood risk-adjusted credit risk scores for loan approvals.
Why is ESG data essential for investors?
ESG data is used by asset managers and investors for market analysis, supporting asset allocation and risk management, and in providing insights into the long-term sustainability of investments in various corporations.